Navigating in the New AI-Driven E-commerce Landscape
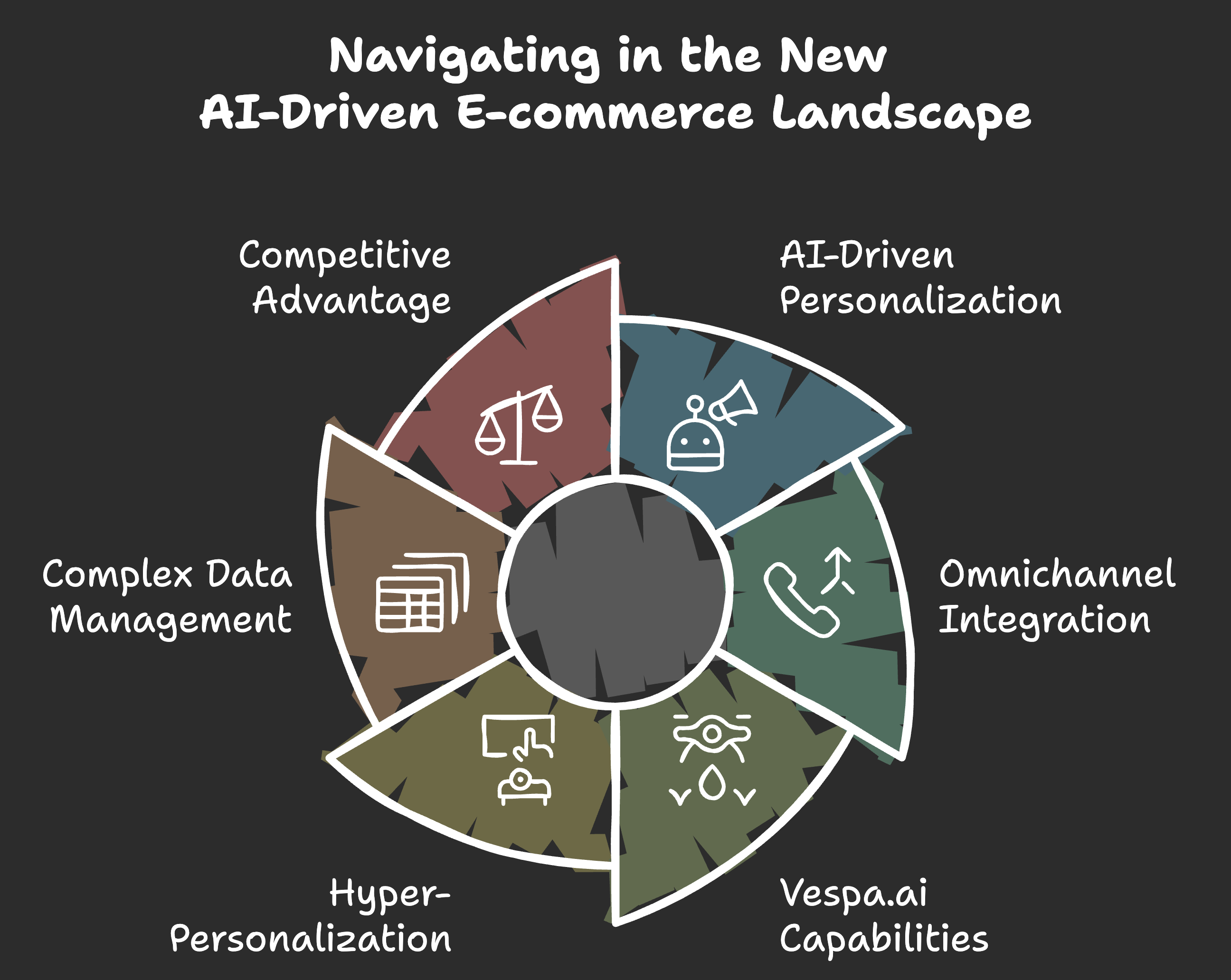
Over the past decade, the e-commerce industry has undergone a remarkable transformation. What began as simple online shopping carts has evolved into complex digital ecosystems powered by data, artificial intelligence (AI), and machine learning (ML). This evolution is driven by consumers’ increasing demand for personalized experiences, real-time interactions, and seamless omnichannel integration. Today’s shoppers expect tailored product recommendations, dynamic search capabilities, and hyper-personalized experiences across multiple platforms, whether they’re on mobile devices, desktops, or even in physical stores enhanced by connected technologies. As a result, emerging trends like AI-driven personalization, contextual search, and recommendation engines have become essential for businesses to meet these expectations and maintain customer engagement.
In this fast-paced e-commerce environment, businesses must adapt or risk falling behind. Companies that fail to leverage AI-driven technologies may experience reduced customer engagement, lower conversion rates, and ultimately a decline in revenue. To stay competitive, it’s crucial for businesses to adopt platforms that offer real-time data processing, scalable infrastructure, and advanced personalization capabilities.
Current Trends & Challenges in E-commerce
Hyper-Personalization and Contextual Relevance
Hyper-personalization involves delivering dynamic content based on real-time analysis of each user’s interactions across various touchpoints or different signals. However, delivering such experiences at scale presents significant technical challenges.
Complex Data and Search Demands
The exponential growth of data in e-commerce has made it increasingly difficult for traditional systems to deliver fast and accurate search results. Consumers expect instant access to relevant products based on complex queries involving keywords, filters, and even image-based searches. Vector search, where high-dimensional data like product embeddings are used, has become critical for improving relevance ranking in searches.
AI and Machine Learning for Competitive Advantage
Leading e-commerce platforms now utilize AI for everything from product recommendations to predictive analytics and customer segmentation. Companies that adopt AI-driven technologies can offer more personalized experiences while optimizing their operations through automation. Those that fail to innovate will not only lose customers but also miss out on the efficiency gains that AI can provide.
Omnichannel Experience Integration
Seamless integration across web, mobile, voice assistants, and physical stores is no longer optional - it’s a necessity. Consumers demand consistent experiences regardless of the platform they use. This requires advanced data synchronization capabilities that most legacy systems are unable to provide effectively. Without omnichannel integration, businesses risk delivering disjointed customer experiences that lead to frustration and churn.
Introduction to Vespa and its Unique Capabilities
Overview
Vespa.ai is a cloud platform designed for building high-performance applications that require real-time search, recommendation engines, ad serving, and analytics at scale. Originally developed by Yahoo!, Vespa has been battle-tested for a few decades across large-scale applications serving billions of users globally. It excels in handling complex queries involving both structured and unstructured data while integrating seamlessly with machine learning models.
Vespa.ai provides modern e-commerce businesses with essential tools to incorporate the latest AI capabilities. It specializes in scalable data serving, classic and vector search, tensor operations, and AI model serving and continuous ML/AI model updates, offering various customizable building blocks. This comprehensive suite enables efficient and accurate data retrieval and data processing, empowering businesses to enhance their platforms with advanced AI functionalities.
The platform’s architecture was optimized over years, supports real-time data processing and querying of massive datasets with minimal latency without the need to move the data around. This means customers receive immediate responses to their queries, and the system can dynamically update and adapt based on user interactions, enhancing the overall shopping experience and business outcome.
By adopting Vespa.ai, organizations can initiate a path of continuous innovation, starting with improved search functionality and advancing toward more sophisticated AI-driven applications. Its exceptional performance, scalability, and adaptability enable substantial e-commerce platform improvements while keeping costs under control. This positions businesses to scale efficiently, maintain operational excellence, and stay competitive in an increasingly data-driven market.
Use Cases of Vespa in E-commerce
-
Personalized Search and Discovery: Vespa’s advanced search capabilities allow businesses to deliver personalized search results tailored to individual preferences, shopping histories, and other relevant customer signals. Vespa ensures customers find relevant products quickly while enjoying a seamless shopping experience by being able to use all search techniques, visual, faceted filtering, or through natural language query.
-
Recommender Systems: Vespa’s support for real-time machine learning enables enhanced recommendation systems that consider customer context, behaviors, and preferences in real-time. This leads to more accurate product suggestions that drive higher engagement and conversions.
-
Inventory Optimization and Dynamic Pricing: Vespa can help businesses develop systems to optimize their sales processes by enabling real-time inventory updates and tracking, dynamic pricing adjustments, and adaptive product rankings based on competitor activity and aggregated data signals. This comprehensive capability ensures that companies remain competitive while maximizing revenue opportunities.
-
Customer Analytics and Ad Serving: With its powerful analytics capabilities, Vespa provides real-time insights into customer behavior. These insights can help to serve dynamic ads which are adjusted well for customers, which may become an additional revenue stream.
-
Image-Based Product Grouping: By combining image embedding models with Vespa KNN (k-nearest neighbors) or ANN (approximate nearest neighbor) similarity search and ranking, it is possible to build efficient, real-time, image-based product groupings. In e-commerce platforms where limited or incorrect metadata is available, this approach can help to easily identify misconfigurations and enable customers to find offers that would otherwise remain unseen.
-
Customer Service and Chatbots: Vespa enables organizations to build comprehensive chatbots which are easily scalable for any customer base size or number of interactions. The fact that Vespa can deliver very high accuracy data retrieval can be used for much better performing RAG and chat discussion relevance and outcome quality, which is essential.
Vespa.ai Users and Their Achievements
Farfetch
At Farfetch, Vespa powers the recommendation system for delivering personalized product suggestions to millions of users in real-time. The platform enables low-latency, high-throughput recommendations by efficiently handling large-scale data and integrating machine learning models directly into its serving layer. This setup allows Farfetch to provide tailored search results and product suggestions, adapting to individual user preferences and behaviors in milliseconds.
Vinted
Vinted transitioned to Vespa to enhance the performance and scalability of their search and recommendation systems. Vespa’s ability to handle complex, large-scale data and support machine learning models natively allowed Vinted to provide faster, more personalized search results. By leveraging Vespa’s real-time indexing and flexible architecture, Vinted improved both the speed and relevance of its recommendations and search functionalities, offering users a more seamless experience when browsing second-hand items. This move also enabled Vinted to better scale with its growing user base while maintaining high efficiency and customization for search and discovery use cases.
Vinted Recommender System
Vinted has adopted the Vespa engine to enhance personalized homepage listing recommendations. Their three-stage recommender system combines explicit user preferences, such as specified clothing sizes, with implicit preferences derived from historical interactions like clicks and purchases. Transitioning to Vespa, Vinted addressed challenges with data management and filtering capabilities by offering robust data handling and the ability to filter items based on metadata, thereby improving the recommendation process. This integration has significantly enhanced Vinted’s ability to deliver tailored content to its users.
I don’t know why I hadn’t looked at this or used this before as much as I do now.” “Actually, Vinted is I think the only app that I use to just browse the main page because the stuff that comes up there is personalized to the user and based probably on my recent searches and recent buys and finds.” “I’ve recently found that I do find myself overnight time scrolling through. Actually, the matches are pretty good, you know, often where I put quite a lot of stuff in my favorites by just looking at that.
_Quote from Vinted.
Spotify
Spotify introduced natural language search for podcast episodes, enhancing discovery by allowing users to search using conversational phrases like “podcasts about space exploration.” Over time Spotify moved the entire search experience and recommendation use cases to Vespa. By leveraging Vespa, Spotify can efficiently process these complex queries, using machine learning models to understand context and meaning, and deliver highly relevant results in real-time. Vespa’s ability to handle large-scale data and support advanced search capabilities ensures that users can find what they look for even if they don’t use specific keywords, making the discovery process more intuitive and personalized.
Spotify Podcast search using Vespa
OTTO
The team at Otto.de, Germany’s second-largest e-commerce platform, embarked on a journey to improve their auto-suggestion functionality, ultimately finding an ideal solution in Vespa. Initially relying on Elasticsearch, they moved through different stages of development, including a custom Python application. However, they sought a more powerful platform for incorporating machine learning and further optimizing their system, which led them to Vespa. With Vespa, the team seamlessly integrated a LightGBM model for re-ranking suggestions, significantly enhancing the relevance of autosuggestions. Vespa also improved performance, reducing response times and eliminating latency spikes, even during peak usage. Additionally, the platform enabled contextualization, allowing suggestions to be personalized based on user search history, creating a more tailored experience. The ease of deployment and flexibility made Vespa an attractive choice. The team could move much of the logic from their Python application into Vespa, enabling advanced features like result clustering. By switching to Vespa, Otto.de was able to enhance the functionality and scalability of their autosuggest feature while incorporating advanced machine learning capabilities and maintaining excellent performance, all in a flexible and cost-effective setup.
Deployment Considerations
Scalability and Infrastructure
Vespa Cloud is designed to handle large-scale applications efficiently, offering features like automatic scaling to adjust resources based on real-time demand. This ensures optimal performance and cost-effectiveness by allocating resources as needed. Its distributed architecture supports seamless scaling, allowing applications to manage increasing data volumes and user requests without compromising performance. Additionally, Vespa Cloud provides continuous deployment and upgrades, enabling applications to evolve and scale smoothly over time.
Data Privacy and Security
Vespa Cloud prioritizes data privacy and security through several key measures:
- Mutual TLS (mTLS) Authentication: All communication between services is protected using mTLS, ensuring that only authenticated clients can access endpoints and that services communicate with trusted sources. (Vespa Blog)
- Data Encryption at Rest: All customer data is encrypted at rest using the cloud provider’s native encryption capabilities (AWS KMS or Google Cloud KMS). (Vespa Cloud)
- Access Control and Service Isolation: Vespa Cloud employs strict access control mechanisms and service isolation to prevent unauthorized interactions between different applications and services. (Vespa Cloud)
These measures collectively ensure that Vespa Cloud maintains a secure environment for data processing and storage.
Integration with Existing Systems
Vespa is designed for easy integration into existing technology stacks through its flexible APIs. Businesses can adopt Vespa incrementally without disrupting ongoing operations or requiring a complete re-platforming effort.
Conclusion
The future of e-commerce lies in leveraging advanced AI technologies like those offered by Vespa.ai. Businesses must embrace innovation if they want sustainable growth amid fierce competition. By adopting Vespa’s powerful platform for real-time search, recommendations, and analytics at scale, companies can differentiate themselves from competitors while driving deeper customer engagement – and ultimately achieving long-term success through continuous innovation.