Customer Engagement: Where the Generative AI Rubber Hits the Road
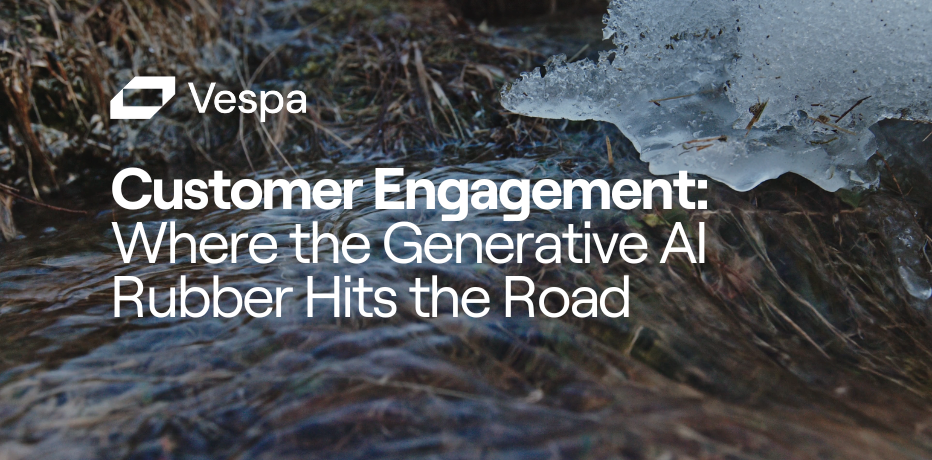
A Missed Sales Opportunity
I’m a big fan of Amazon, but I am always surprised when they recommend products similar to those I just purchased. How many kitchen sink organizers does a home need? I recently visited the UK retail institution marksandspencer.com, looking to spend money, so I hit “inspire me” but was surprised they recommended a slew of women’s clothes. While the beautiful broderie anglaise, the summer sleepwear, and the oh-so-chic linen were very nice, me being a “traditional bloke from Yorkshire,” was not inspired to make a purchase–a missed sales opportunity. Or maybe M&S knows more about me than I know myself!
In the blink of an eye, genAI has leaped from the pages of an Arthur C. Clarke novel to become a component in e-commerce–revolutionizing product search, personalization, and customer engagement. However, my online experiences suggest we’re just beginning this journey. Gartner’s infamous hype cycles back this up. Their Hype Cycle for Generative AI, 2023, positions it as nearing a peak of inflated expectations, meaning we’re likely in for a letdown soon. The good news? After navigating the trough of disillusionment, users will adapt to the technology, traverse the slope of enlightenment, and eventually reach a plateau of productivity.
Disillusionment Factors
In my opinion, the causes of this gap in expectations vs. reality include:
- Lack of experience: This disruptive technology is new, requiring time to learn best practices, gain confidence, and understand its full potential.
- Vendor hype: AI is the latest trend, making it difficult to distinguish between genuine capabilities and exaggerated claims.
- Search accuracy: GenAI outcomes depend on good search, which involves analyzing structured and unstructured data, sophisticated reranking, and machine learning to refine results.
- Scalability and performance: Few genAI platforms can handle the scale of thousands of concurrent queries. Even less can do so without costly GPUs, forcing a cost vs. accuracy tradeoff.
E-commerce is the CMO’s killer app for genAI: it’s customer-facing, mission-critical, and directly impacts revenue. Since genAI is an incremental add to well well-functioning system, it’s a proving ground for genAI. Recommendation, search and personalization have been critical for customer engagement long before genAI was ever spoken about. Amazon led the way at the turn of the century, and since then, we’ve seen the addition of big data, cloud computing for scale and elasticity, and machine learning to predict the next best action (NBA). GenAI is simply the next logical step in this ongoing evolution.
Garbage In, Garbage Out
The success of genAI hinges on the quality of the data it uses—garbage in, garbage out, as the saying goes. While many believe it’s all about vectors, these high-dimensional data representations are just part of the picture. To boost accuracy and relevance, it’s essential to tap into the broadest possible information pool. This includes structured data, like text and keywords, and unstructured data, like product reviews (but only from similar-minded customers).
Multi-phase reranking is critical, delivering a comprehensive method that continuously refines search and recommendation results. It adapts to constantly changing data inputs and applies custom business rules, ideally leveraging ColBERT (Contextualized Late Interaction over BERT), an advanced model for efficient large-scale information retrieval. Machine learning is key here, optimally combining signals from many data sources. Ultimately, high-quality data retrieval drives high-quality outcomes in large language models (LLMs).
GenAI is the icing on the cake. For example, Vespa.ai can use it to enhance information processing and delivery. When a user searches for something, Vespa.ai can use genAI to filter, extract, and synthesize the surfaced information. This enables it to generate relevant content that directly answers the user’s query, such as summaries, recommendations, or detailed explanations based on the available data.
Managing One-to-One Relationships as Scale
Scale and performance are essential for e-commerce platforms to handle high traffic, provide excellent user experiences, delight captive customers, operate efficiently, support growth, manage data effectively, and serve a global audience. Given the seasonality of retail, elasticity is essential.
It’s hard not to discuss this without getting into a product architecture pitch, but the blog Unlocking Ecommerce Growth: The Power of AI in Personalization and Recommendation details how Farfetch uses Vespa.ai to engage four million customers in their e-commerce platform.
Vespa achieves high performance and scalability through its distributed architecture, efficient query processing, and advanced data management. By distributing data and queries across multiple nodes in a cluster, Vespa ensures load balancing and fault tolerance, handling large data volumes and high query throughput without specialized processors like GPUs. Horizontal scaling allows adding nodes as needed, effectively managing growing datasets and higher query rates.
Efficient query processing is crucial to Vespa’s performance. The platform is optimized for low-latency execution with advanced optimization techniques and in-memory data structures, reducing access times. Real-time data ingestion and updates provide immediate availability of new or modified data. Vespa’s powerful query language supports complex queries, filtering, and ranking, enabling sophisticated searches. Additionally, advanced ranking algorithms use machine learning models to deliver relevant, high-quality search results.
Summary: Well Worth the Hype!
As for vendor hype, I’m a tech company CMO prone to say “AI” a lot (note to self: maybe tone it down a bit!) However, I felt vindicated by reading this excellent Redmonk blog: AI and the Future of Search. GenAI is a game-changer for enhancing processes, though, for now, it shines brighter in some use cases than others.
And that’s all I have to say about that!